Latent Space Podcast 4/28/23 [Summary] - Mapping the future of *truly* Open Models and Training Dolly for $30 — with Mike Conover of Databricks
Explore the future of open models with Mike Conover of Databricks. Dive deep into Dolly's creation, its transition from 1.0 to 2.0, & the influences behind its development. Ep.9 touches on model infrastructure, Databricks' vision, & more. #AI #OpenModels #Dolly
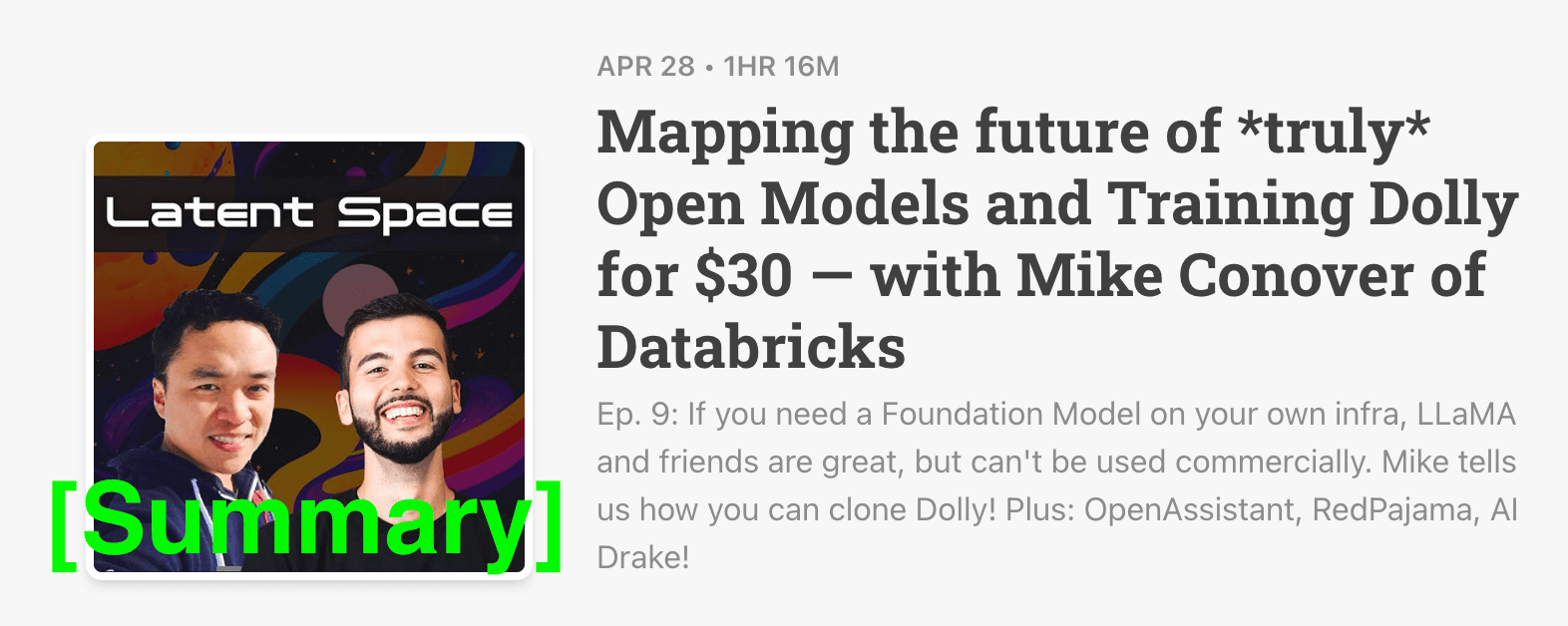
Original Link: https://www.latent.space/p/mike-conover#details
Summary
In this episode of the Latent Space Podcast, hosts Alessio Partner, CT and Residence and Decibel Partners, Joan Bama, and swyx Brighter welcome guest Mike Conover.
Introduction of Mike Conover: Mike is a staff software engineer at Databricks. His educational background is rooted in complex systems analysis at Indiana University, where he performed analysis of clusters on Twitter. He has worked at LinkedIn, where he focused on the homepage news relevance, later moved to SkipFlag, an enterprise knowledge graph, and then transitioned to Workday after its acquisition. At Workday, he took on the role of the director of machine learning engineering.
Personal Insights: Mike shares his love for exploring off-trail in the backcountry, drawing a parallel between understanding topographic maps and the way he looks at high-dimensional spaces in machine learning. He also enjoys camping trips and archery.
About Dolly: Dolly, a project from Databricks, quickly became a significant open-source sensation. In its initial version, Dolly 1.0 was based on the GPT-J model with 6 billion parameters and was trained using the alpaca training set. The choice to use GPT-J instead of LLaMA was due to its accessibility and familiarity.
Development of Dolly: Mike discussed the creation of Dolly and how it was driven by his interest in how information moves through networks of people. The inspiration for Dolly was to improve the developer experience, making it more intuitive and interactive.
Dolly 2.0: A significant upgrade was introduced with Dolly 2.0, which has 12 billion parameters. This version was based on the Elu model family. Instead of using the alpaca training set, Dolly 2.0 was trained with a new dataset created by Databricks employees. The shift to a more personalized dataset came as a bid to make the tool more comprehensive and user-centric.
Throughout the podcast, Mike emphasizes the passionate drive behind Databricks projects and the collaborative spirit that allows for rapid innovations like Dolly.

Exploring AI’s Evolution: From Data Synthesis to Geopolitical Negotiations
Gamifying Instruction Tuning:
Discussing the FLAN dataset, which has thousands of tasks, it's highlighted how it prevents models from overfitting as it requires generalization across tasks.
The dataset's brief response structure makes models generate shorter results.
They touched on the leaderboard's gamification and how certain participants tended to dominate contributions, likening it to the "long tail" distribution observed in human systems.
They emphasize the importance of genuine usefulness rather than just perceived performance.
Summarization - Thumbnails for Language:
Summarizing data is challenging due to its need for synthesis, especially when the source material is lengthy.
They delve into the idea of "thumbnails for language," drawing a parallel with how the visual cortex processes images quickly. They discuss how AI might shape the way we process large text chunks, optimizing comprehension.
They jokingly mentioned using emojis as potential textual thumbnails.
CICERO and Geopolitical AI Agents:
They explore the potential for AI in resolving geopolitical disputes. The Cicero paper from Meta illustrates this, where AI was used in a negotiating game simulating diplomacy.
They envision nation-states deploying AI systems that can find non-exploitable, game-theoretically optimal solutions to geopolitical problems.
Additionally, they speculate on AI's potential to personalize information compression for individuals to maximize comprehension.
Datasets vs Intentional Design:
Comparing the traditional design of items like jet turbines with AI, the conversation leans into the discovery of latent capabilities in models.
The Pithia Suite is introduced as a matrix of model checkpoints and sizes, exploring how behaviors evolve during training.
There's discussion about reproducing the LLaMA dataset, especially concerning the challenges of managing such large datasets. They emphasize the potential for more intentional AI training in the future, tailoring models for specific tasks.

Exploring the Intersection of Biology, Classic NLP, and Generative AI in Modern Tech Development
Biological Foundations of AI: The discussion revolved around understanding AI's development using a biological approach. The idea of "speed running evolution" was presented, emphasizing AI's rapid development. The differences in the evolution of artificial and biological life were also discussed, citing Richard Dawkins' "bio morphs" as an example. These bio morphs are vector-art-looking insects where parameters like the number of legs, antennas, etc., are recombined using genetic algorithms, offering a visual representation of synthetic evolution based on aesthetic preferences.
Training and Adapting LLMs (Large Language Models): The conversation shifted to how businesses could adapt technologies like Dolly. While some might think Databricks’ work with Dolly is merely to showcase capabilities, the primary intent is to assist businesses in understanding and implementing these technologies effectively. The difference between an LLM’s generic outputs and a business-specific requirement was highlighted, like the difference between writing a generic moody letter and crafting a business-centric message. The process of instruction tuning and the importance of understanding model size relative to the task was touched upon.
Evaluation and Efficiency: The discussion emphasized the importance of effective evaluation benchmarks. Although models like GPT-J and Dolly might score similarly in benchmarks, their qualitative outcomes are vastly different. The challenge lies in measuring a model's desired behavior, especially in enterprise contexts. The idea of human-in-the-loop feedback and active learning was introduced, emphasizing the importance of humans guiding AI systems.
Balancing Old Techniques with New: There's still value in classical machine learning techniques. Not everything needs a generative AI approach. For instance, named entity recognition or multi-class classification for categorizing customer support tickets are simple yet valuable tasks. The speed of inference was discussed, noting that Databricks' system is notably faster than many others. Classical ML models could be used in conjunction with generative models for efficiency, challenging the notion that agents will only communicate with agents.
Future and Best Use Cases of LLMs: Generative AI remains an exciting field with many potential branches yet to be explored. However, there's a belief that a blend of older techniques with new ones might yield optimal results. Generative models excel in tasks only they can achieve, like generating customer support replies in a company's specific tone and voice. This could revolutionize how businesses approach problem-solving, making tasks that took machine learning teams weeks to complete doable in minutes. However, the true cost-saving potential remains to be seen.

Understanding Dolly's Cost-Effective Training on Databricks
Dolly, a model developed on Databricks, garnered attention for its cost-effective training price point of just $30. This intriguing value was achieved by training the original Dolly on Databricks clusters, a platform that proved efficient for multi-node distributed fine-tuning. Utilizing Databricks allowed the researchers to train Dolly in less than an hour for 50,000 records, demonstrating both the power and efficiency of the platform.
Open-sourcing has been integral to the success and approach of Databricks, with its founders holding strong beliefs in communal benefits and mutual success. They've consistently released technologies to the public that most would view as valuable IP. This has created an ecosystem where innovation thrives and the community reaps mutual benefits.
Moving into the domain of "LM ops", which refers to language model operations, there's a recognized need for new and efficient tools. Developers require platforms that not only provide quantitative benchmarks but also qualitative subjective benchmarks, along with human-in-the-loop feedback. Databricks aims to address this gap by developing solutions that cater to the evolving requirements of AI developers.
Despite available tools like Prompt Layer, a significant need remains for a comprehensive tool that merges speed, efficiency, and user-friendliness, offering an improved alternative to traditional methods like spreadsheets.

AI, Spreadsheets, and the Evolution of Productivity: A Glimpse into Future Workspaces
In a discussion on AI's role in modern technology:
The speaker references an investment called "Quadra" which seems to be a tool similar to Google Sheets but has features such as web assembly, canvas, and the capability to use languages like Python, SQL, and Scala. The value proposition is to improve the utility of sheets-like platforms by integrating AI-like template filling without obstructing experimentation.
The conversation then shifts to AI's role in workplace productivity. The impact of AI is compared to the introduction of spreadsheets in the 1980s. Professionals initially resisted spreadsheets but later embraced them as they realized that the technology eliminated tedious aspects of their jobs, allowing them to focus on more valuable tasks.
The topic of AI-driven writing is touched upon. The speaker believes that although there's stigma around AI-generated content now, in the future, AI might be trusted to draft emails or negotiate contract details on behalf of users.
Mention is made of "OpenAssistant," "CerebrasGPT," and "Red Pajama" - each being different AI projects or technologies. The speaker praises OpenAssistant for its open data set and suggests it will significantly impact future AI endeavors.
A method for paraphrasing prompts is discussed which involves translating a prompt to another language and back to get a differently worded prompt.
"Red Pajama" seems to be related to a paper named "LLaMA" which uses multiple data sources, including common crawl and Wikipedia, to train its model. The complexities and challenges of using such large datasets for training are discussed.
Note: This summary encapsulates the primary themes and topics discussed in the transcript and streamlines the content for easier understanding.

Exploring the Versatility and Evolution of AI Models: Dolly, OpenAI, and Self-Reflection.
Dolly's Edge Over OpenAI GPT The distinction between "open" and commercially usable tools was discussed, emphasizing how Dolly provides clarity in its licensing for commercial use. Many businesses found it hard to use Dolly 1.0 due to its training data sources. However, Dolly's recent advancements are clear and designed for business applications, from processing public web data to managing customer support tickets. One significant value proposition is that businesses can confidently build on Dolly without licensing ambiguities, and this clarity has garnered a positive response.
Open Source Licensing for AI The shift from traditional open-source licensing that revolves around code to newer paradigms that emphasize the importance of the model's weights was explored. The licensing regime needs clarity, and while external validations, like the Open Source Initiative, might be ideal, clarity in semantics is paramount.
The Value of Open-Sourcing AI Models Open-sourcing AI models can help in addressing challenges more comprehensively. By exposing these models to a wider audience, especially those who can critically evaluate them from different perspectives, like ethics or security, we can get a more holistic understanding of potential pitfalls. The alternative is to blindly trust internal teams, which may not always have a comprehensive view.
Moving Between Models Transitioning between models, even iterations of the same model (e.g., GPT-3.5 to GPT-4), can produce varied behaviors. A future product class could involve meta-models that evaluate the outputs and facilitate such migrations. A need for an infrastructure that aids in moving prompts from one model to another was also highlighted.
Learning in a Simulation Drawing parallels to real-world experiences, the discussion veered towards the concept of models facing repercussions. Just as humans learn from mistakes, models currently don't have a mechanism to "suffer" consequences from the suggestions they make. There's intrigue around how future models might evolve if they face "repercussions" for incorrect suggestions.
Model Reflection & Self-Criticism One fascinating concept touched upon was the self-reflexivity of models. AI models, such as those from Lang Chain, can judge the correctness of their generated content when presented with it, post-generation. This "reflection" allows the model to attend to the entirety of its generated content and judge its validity. The hope is that models can become more accurate by playing against themselves, similar to how AlphaGo improved by playing against itself.

Lightning Round Discussion on AI's Impact
Favorite AI Product: Google Maps was highlighted due to its ability to adaptively visualize information, integrate machine learning, and personalize user experiences. The speaker uses it reflexively and appreciates the unseen design that makes the application so intuitive.
AI in Advertising: The potential for advertising on Google Maps was mentioned, emphasizing its prime real estate for businesses.
Influential AI Communities: The hugging face group was commended for simplifying complex processes in the AI industry. Their commitment to open source projects such as Transformers and diffusers was appreciated.
Future Surprises in AI: The imminent possibility of AI-generated music and the rise of "character AI" was discussed. There's potential for high-fidelity avatars that represent individuals' beliefs and intents based on their past communication. This development may challenge societal norms and perceptions.
AI in Music: AI's capability to produce music that feels organic was discussed, with reference to a song by Kanye West and Weekend. The implications for music labels and the blurring distinction between true creativity and formulaic production were touched upon.
Desired AI Applications: The potential for AI in optimizing outdoor experiences was emphasized. The U.S.'s vast public lands could be better utilized with AI-guided exploration. Another idea proposed was the use of AI for swarm management – using multiple sensors and inputs from various agents to, for instance, monitor backcountry trail conditions.
The conversation revolved around the ever-expanding applications of AI, from the mundane to the profound, and the impacts of these advancements on society, creativity, and business.