Latent Space Podcast 8/4/23 [Summary] Latent Space x AI Breakdown crossover pod!
Join AI Breakdown & Latent Space for the summer AI tech roundup: Dive into GPT4.5, Llama 2, AI tools, the rising AI engineer, and more!
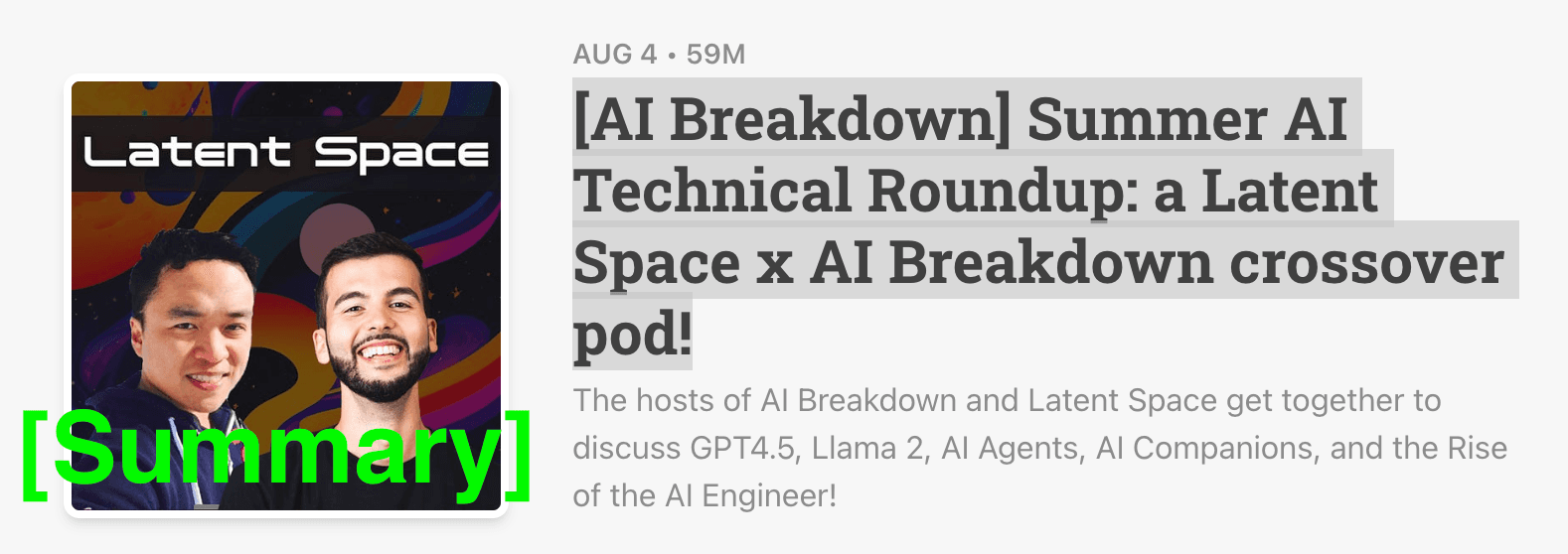
Original Podcast Link:
[AI Breakdown] Summer AI Technical Roundup: a Latent Space x AI Breakdown crossover pod!
Podcast Summary
[0:00 - 11:51]
Agenda and Introduction:
An initial exchange of pleasantries with participants expressing their excitement about the session.
Recognition that the AI audience is diverse, comprising both technical experts in the field and those who are non-technical but keenly aware of the rapid developments in AI. There's a strong interest in understanding the recent trends and major events in AI over the past month, particularly in July.
Discussion of Code Interpreter from OpenAI:
Code Interpreter is initially presented as a chat GPT plugin but has functionalities closer to GPT 4.5. The significant difference it offers from previous models, like the chat GPT, makes it worth noting.
The launch of the Code Interpreter wasn't heavily publicized by OpenAI but has garnered attention for its capabilities, which seem to surpass those of GPT-4. Some speculate it's a step forward but not officially labeled to avoid potential controversies.
Enhancements in GPT 4.5:
Code Interpreter handles non-coding queries and diversifies ChatGPT's coding support.
While previous models struggled with direct mathematical questions due to how numbers are tokenized, the Code Interpreter overcomes this by focusing on code that can answer such questions.
It caters not just to developers but also non-technical individuals, offering solutions to problems akin to a junior developer or analyst.
The significant distinction between GPT 4.5 and its predecessors is its capability to handle coding tasks effectively.
Shift in AI Development Focus:
The shift from simply using larger datasets for training to focusing on inference time optimizations marks a change in AI development. This is believed to be the direction towards AGI (Artificial General Intelligence).
OpenAI's origins in gaming AI and the use of reinforcement learning environments hinted at this trend, where the AI would vary its inference time based on problem complexity.
GPT 4.5, particularly with the Code Interpreter, is viewed as an evolution that incorporates tools to enhance its capabilities. It is compared to humans evolving and then using tools to achieve tasks that were previously challenging.
Overall, the development and functionality of Code Interpreter represent a shift in AI capabilities, allowing the model to not just be bigger but smarter in its operations.
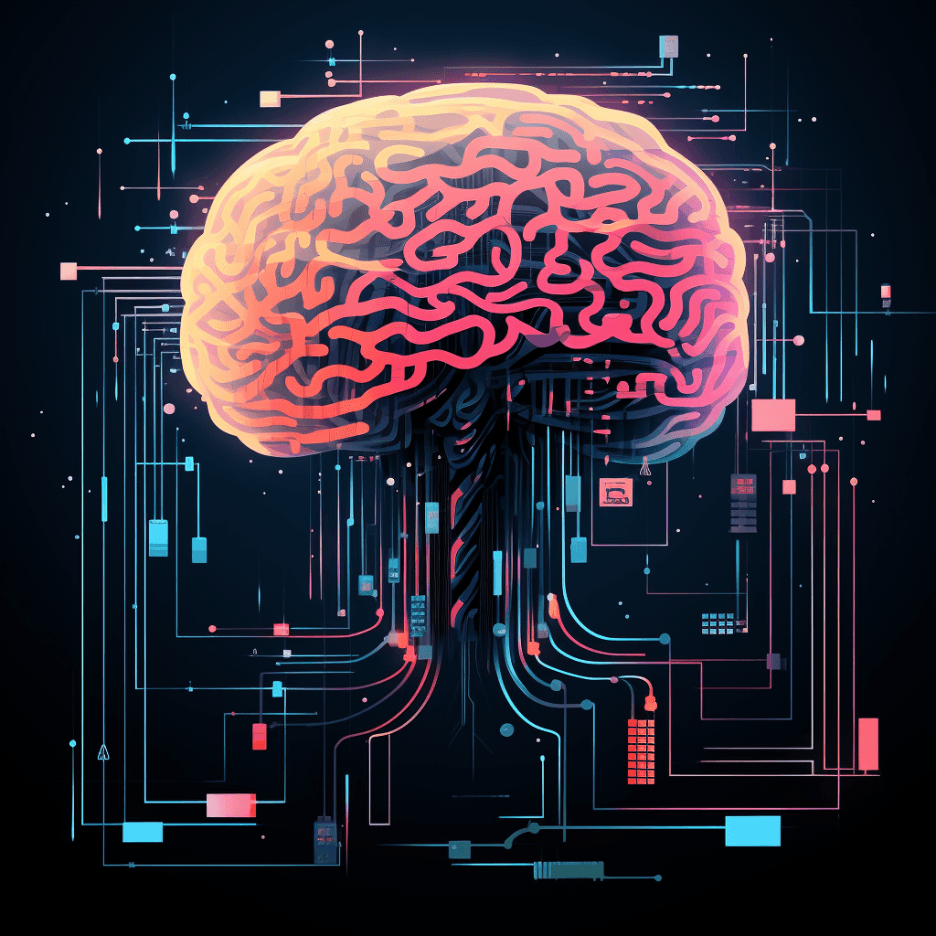
[11:51 - 19:36]
Performance concerns about GPT4:
There's been speculation and research indicating that GPT4's performance might have deteriorated over time or has been intentionally nerfed.
Challenges in Evaluation:
Evaluating the effectiveness of models like GPT4 is difficult, especially for open-ended tasks.
Examples of evaluation include assessing if code suggestions in programs like Co-pilot are maintained over time.
There's ambiguity in evaluations: e.g., changes in formatting might not necessarily indicate the model's inefficiency.
With reinforcement learning, it's hard to determine what the model is anchoring on, leading to inconsistencies.
Pressure on OpenAI:
OpenAI faces the challenge of ensuring safety and providing consistent outputs, especially as they roll out updates.
There's a suggestion to "version lock" the model to better understand its evolution and changes.
Transition of OpenAI:
OpenAI has evolved from a research lab to a product/infrastructure company, creating challenges in balancing research evolution and product reliability.
There's an identified communication challenge within OpenAI: one spokesperson suggests models are static, while another suggests they're updated frequently. This creates confusion among users.
There's a comparison to Facebook's evolution, where even small algorithmic changes had immediate feedback and ramifications, but OpenAI doesn't receive the same immediate feedback.
Feedback Challenges:
Unlike platforms like Facebook, which could gauge success through metrics like click-through rates, OpenAI has limited immediate feedback mechanisms.
Custom Instructions Feature:
OpenAI introduced a "custom instructions" feature that allows users to personalize GPT-4 more effectively.
This feature existed in OpenAI's playground and other platforms like Perplexity AI and Character AI. So, while significant for GPT users, it's not a brand-new capability in the AI chatbot sphere.
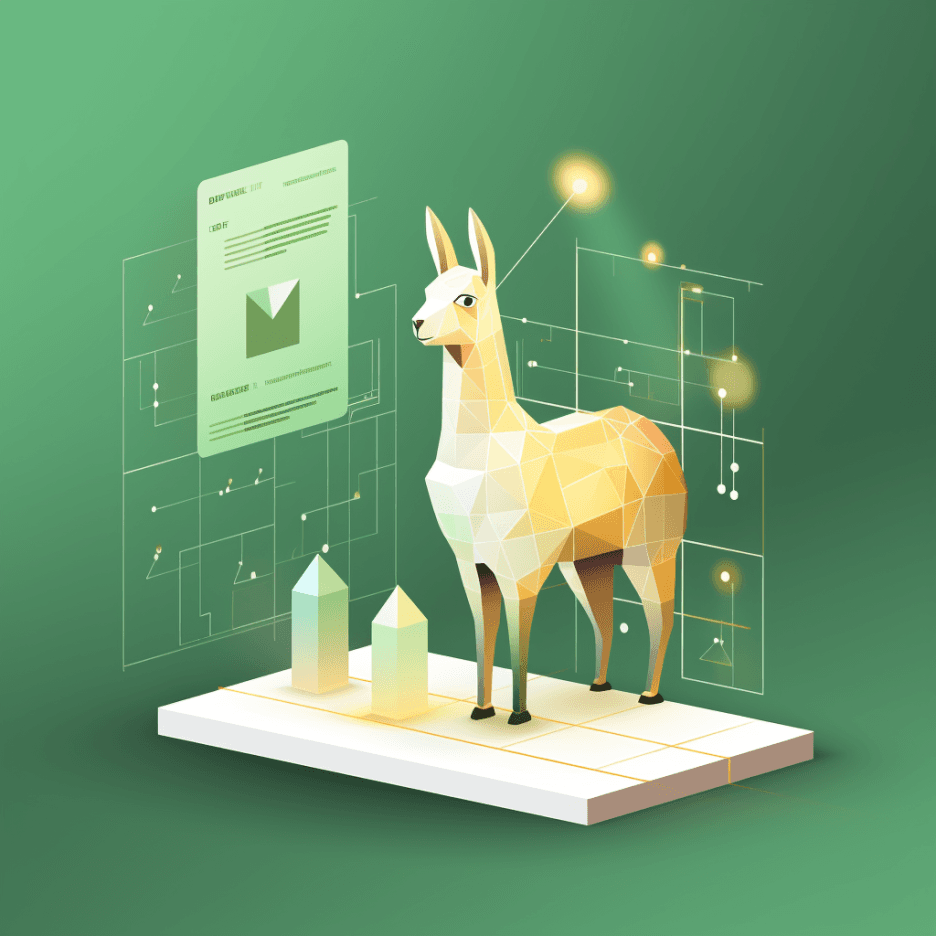
[19:36 - 32:04]
Facebook's release of Llama 2 represents a significant step in AI development, symbolizing a shift in the balance between open-source and commercial models. Here's a comprehensive summary of the details and implications from the transcript:
Technical Overview:
Llama 2 is described as the first fully commercially usable GPT-3.5 equivalent model. It can run on private infrastructure, opening new possibilities for government, healthcare, and financial sectors, and users can fine-tune it with full control over the internals.
Open-Source Debate:
Despite not being fully open-source, Llama 2 has been generously shared with the community. The debate around its status has led to reflections on the need for better labeling and definition for models like this. An estimated $15 to $20 million has been effectively donated to the community, with many building on top of it.
Shift in Value:
Llama 2 reflects a change in AI's relative value, with data becoming more valuable than compute. It marks a reversal in open-source culture, where the sequence of openness has shifted. There's a growing trend to restrict data while still providing access to models, indicating a more protective stance towards proprietary data.
Legal and Ethical Considerations:
There's a growing intrigue and concern around regulatory pressure and copyright issues in the AI space. Instances of training on copyrighted data like books have stirred debate on Twitter, highlighting the blurry lines around what constitutes fair use.
Impact on Business and Development:
Llama 2 has significantly impacted the evolution of the AI space and business strategies. Companies are now less reliant on startups for AI solutions, as technical teams can spin up their versions using open-source tools. It has raised the bar for what vendors must offer, shifting competition towards other areas of AI application production.
Open-Source vs Commercial Models:
The discussion also brings to light the subtle nuances between truly open-source models and those that are labeled as such but come with certain restrictions. The conversation around Llama 2 has served as a focal point for a broader conversation about how the AI community navigates the balance between openness and commercial interests.
Cultural Shift:
There's a cultural shift in how open-source models are handled, with a focus on functional use over strict definitions. The availability of tools like Llama 2 has changed the landscape, demanding more from vendors and reshaping how both startups and large companies approach AI development.
In conclusion, the introduction of Llama 2 has ignited conversations and changes that reach far beyond its technical capabilities. It has become a symbol of the evolving dynamics in AI, marking a period of transition where the boundaries between open-source, commercial interests, regulation, and ethics are being actively explored and redefined.
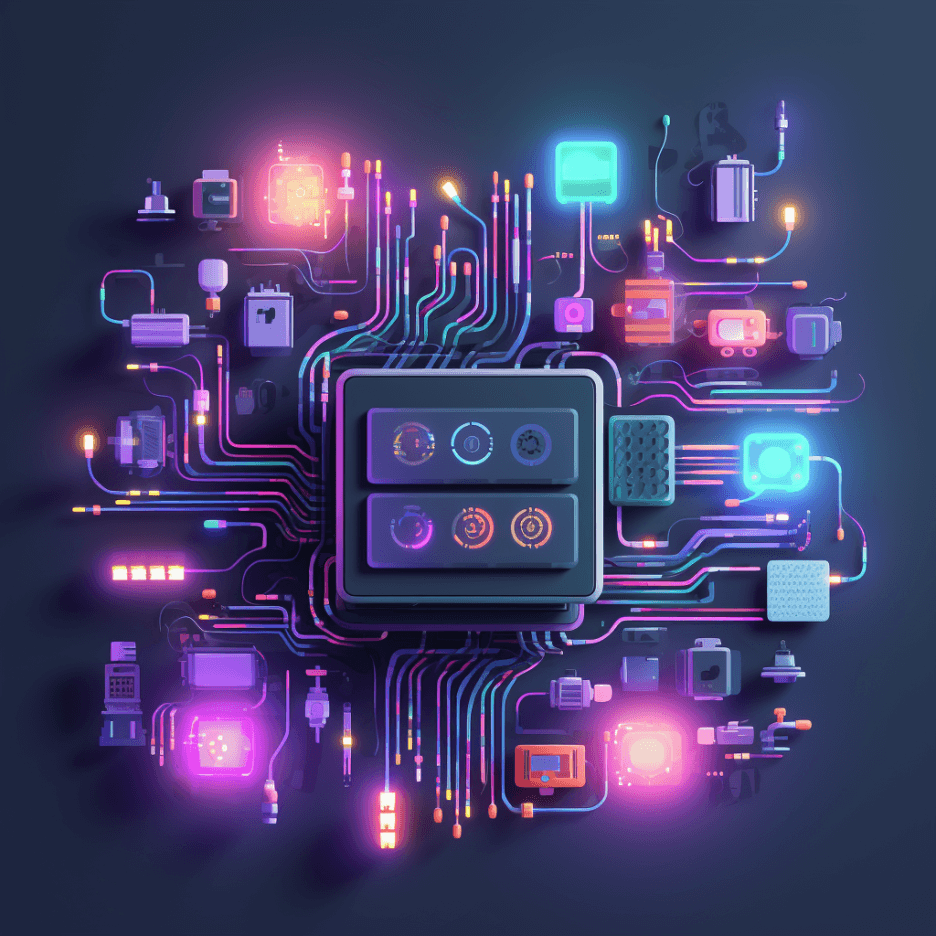
[32:04 - 44:40]
Perspective on AI developments.
Highlighted Developments:
Context Window Expansion: A major change that allows for improved handling of data.
MLC Team's Experiment: The team wrapped llama two to run on MacBook GPUs. This is interesting because it bridges the gap between using token-based AI services and unlimited local usage.
Open Source Creativity: Open Source offers unpredictable potential. The "AI girlfriend economy" is a booming, less-talked-about sector with millions of users. It also brings forth debates on societal perspectives of such technology.
Significance of AI in Relationships:
AI relationships might play a crucial role in addressing loneliness in the future, potentially reducing adverse societal events.
A prediction is made that future generations will find AI relationships normal, leading to generational debates.
Matthew McConaughey discussed the idea of computers assisting humans in interpersonal relationships. These AI tools can help individuals learn and better their interaction with others.
Discussion on Claude 2 & Anthropic:
Claude 2: Considered significant for its longer context window and its utility in aiding developers.
Anthropic: Positioned as a safer alternative to OpenAI, it has made a mark in the competitive CI landscape. While it has been overshadowed by giants like OpenAI, there's a growing appreciation in the developer community for its potential.
Usage Recommendations: Suggestion made to run chats side by side on platforms like Tragicia, Claude, and Llama two to derive maximum benefits.
Context Limitations: There's a discussion on whether too much context might be counterproductive. Models might retrieve data better from the start or end of a context window, but information in the middle might get lost.
The conversation delves into recent AI developments, their implications, and societal perspectives on AI's role in human relationships.

[44:40 - 58:37]
Google Bard Updates:
Mid-month, Google Bard released several updates focused largely on business.
Added support for more languages.
The main feature they highlighted was advancements in image recognition, signaling a move towards multimodality.
Some participants feel Google needs to enhance product level offerings, suggesting integrating Bard with tools like Google Maps.
Despite Bard's efforts in multi-modality, there's been criticism, with some feeling the updates aren't as innovative as they should be.
Bard did, however, achieve a general multimodal availability before GPT-4, where one can upload an image and have Bard describe it.
Some feel Google's consistency in product updates is lacking, referencing Google Adobe's discontinuation and other failed promises.
AI Trends in Developer Community:
Interest in auto GPT-like systems, mentioning GBT Engineer and Multi-on.
Agent technology hasn't died down; there's a push towards making agents more usable.
A rising trend of evaluation companies, which monitor the success of AI models and versions.
There's a shift from generic agent models to verticalized agents, combining industry-specific knowledge with AI capabilities.
SDXL, a text-to-image technology, has been on the horizon but hasn't gained the traction it should have.
Character AI is becoming popular, potentially laying the foundation for AI-native social media platforms. These platforms could serialize personality and intelligence, perhaps hinting at "mind uploading."
Reports suggest Meta (formerly Facebook) might introduce AI personas.
Predictions for August 2023:
Expect more public discourse on open-source models being used in production environments.
Despite current perceptions, some big companies might be transitioning from OpenAI models to open-source models.
August might be a preparation month, with bigger announcements coming later in the year.
The discussion appears to revolve around the rapid advancements in AI, with a specific focus on Google Bard's updates and the broader trends in the AI development community. There is an evident excitement and anticipation for what's to come in the AI landscape, especially as it begins to merge with other industries and consumer applications.