Latent Space Podcast 4/21/23 [Summary] - AI-powered Search for the Enterprise — with Deedy Das of Glean
Ep.8: Dive into AI in enterprise search with Deedy Das of Glean. Unpack challenges in creating an AI search giant, Google vs ChatGPT comparisons, AI infrastructure intricacies, spotting AI-generated text, and why businesses need more than just Document QA.
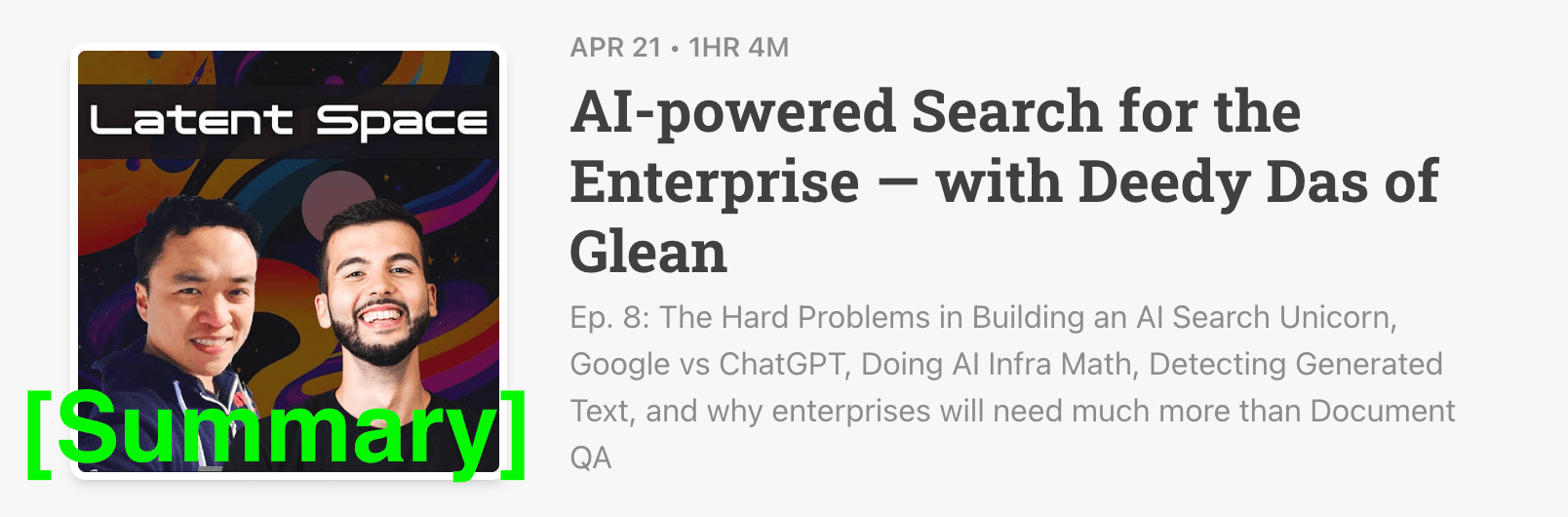
Original Link: https://www.latent.space/p/deedy-das#details
Summary
Introduction Alessio from Decibel Partners welcomes listeners to the Latent Space Podcast, introducing his cohost Swyx, and their special guest Deedy Das from Glean.
About Deedy Das Deedy, who prefers to go by this name over his formal name Debarghya, shares his professional journey:
Completed his bachelor's and master's in Computer Science from Cornell.
Worked at Facebook and Google, specializing in search.
Led a sports team with a focus on cricket.
Transitioned to Glean, contributing to its evolution into a search unicorn that optimizes intelligent search for workplaces. Outside of his professional life, Deedy is an avid reader, particularly of fiction. He praises the book "The Three Body Problem", appreciating its unique blend of alien fiction with physics and its contrast to Western literature.
The Origin and Evolution of Glean Deedy explains that Glean's inception wasn't about creating a product in search of a problem; instead, it aimed to address a significant issue prevalent in many companies. He references Google's internal tool, MoMA, which indexes various internal resources, suggesting that many Google employees miss this functionality upon leaving the company. Glean's purpose was to address the "information retrieval problem" many faced in their workplaces. Historically, the concept of enterprise search isn't new, but technological advances, the proliferation of SaaS apps, and the increasing importance of remote work have made Glean's solution timely and relevant.
From Syntactic to Semantic Search The conversation then shifts to the changing nature of web search: from being syntax-driven to now leaning towards semantic understanding. Glean aids in transforming the enterprise experience by focusing more on the intent behind questions rather than the specific wording or syntax used. For Glean to remain impactful, Deedy believes it's essential not just to offer search functionality but to serve as a comprehensive employee portal, providing features like Go Links, feeds, and mentions, which amalgamate notifications across different platforms like Slack, Jira, and GitHub.
In essence, Glean is bridging the gap in information retrieval in modern workplaces, integrating the convenience of intuitive search with the comprehensive offerings of an employee portal.

The Evolution of Employee Portals and the Shift from Google to AI-Powered Search
Challenges of Employee Portals Like Glean:
The adoption of employee portals is not widespread because selling them involves addressing less tangible benefits like productivity, which doesn't fit the common enterprise sales metrics of increasing revenue or cutting costs. While tools like Glean enhance productivity, showcasing a direct monetary value remains a challenge.
Enterprise Search Technology:
Although buzzwords like "AI-powered" and "vector search" are prevalent, it's essential to realize that user experience matters more than the complexity of the technology. Glean, while utilizing advanced technology, prioritizes the meticulous tuning of its ranking algorithm and takes a hybrid approach combining core IR signal, synonymy, query accentuation, and vector search. Personalization plays a pivotal role in their system, ensuring that users receive relevant search results based on their role and interactions.
Glean Chat and Future Products:
While Glean is always exploring new technologies and products, no specific confirmation about a "Glean chat" was given.
Google vs. ChatGPT:
The user shared their personal inclination towards ChatGPT for many queries, emphasizing that it offers answers to questions one wouldn't typically think the internet could address. However, while ChatGPT is efficient in specific areas, like answering rare or "tail queries", Google is superior in providing comprehensive responses and navigating to relevant resources. There is a decline in traffic on platforms like Stack Overflow, possibly indicating a shift in users seeking technical answers. Finally, the shift of enterprise product search away from Google might impact its AdWords revenue, although the implications of this weren't elaborated upon.
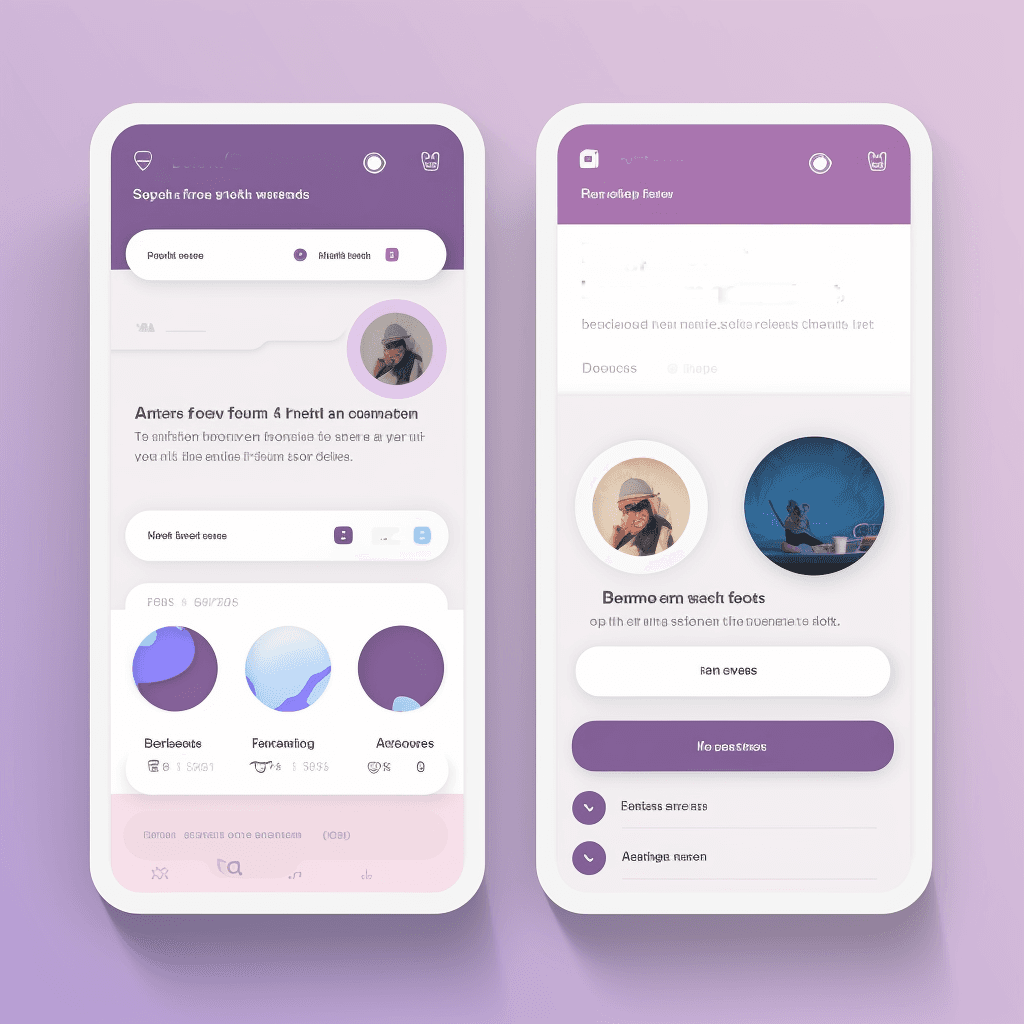
The Future of Search: Freshness, Ad Revenue, and AI
Freshness in Search:
Large language models currently can't rapidly index new information, which Google labels as "freshness."
Retrieval-augmented generation is a workaround, utilizing search results in the background to generate a response. Bing currently employs this method.
Ad Revenue Implications:
Google's predominant revenue comes from placing ad links above search results.
Chat interfaces disrupt this model, as users typically don't click in chat.
A shift from the conventional search to chat might endanger the web ecosystem. Websites might not see the incentive to exist if they're just sources for AI to train on.
Shifting from ad revenue to subscription-based models may not yield similar profits.
Latency Concerns:
Latency affects user engagement; more latency generally leads to less engagement.
However, technological improvements over time are expected to reduce latency.
Google's LamDA model, for instance, employs tools for factual, deterministic information and modifies its response accordingly.
Accuracy and Memorization:
GPT-4's accuracy is primarily due to memorizing facts rather than understanding context or current events. Its factual recall has limitations.
For instance, it can recall data pre-2021 but struggles with events after.
Tool Use and LLMs (Large Language Models):
There's currently a lot of experimentation in how LLMs are used.
Examples like "react" and "tool form" are essentially trial methods that have stuck.
LLMs can also be used to generate synthetic data to bootstrap smaller models, a process that can greatly reduce development times.
Other AI Search Tools - Perplexity and Neeva:
Both products aim to synthesize search results.
Their reliance on existing indexes like Bing, combined with unclear monetization strategies, raises concerns about their long-term viability.
In essence, while AI and search interfaces like chatbots present promising avenues for user interaction, there are inherent challenges tied to information freshness, revenue generation, response times, and the accuracy of results.

Navigating the Future of AI: Challenges, Opportunities, and Imagination
The Challenge with Document QA and LM Startups
The current AI landscape, particularly concerning Language Models (LMS), reveals that while excitement exists around new technologies, there's an over-emphasis on solution-first approaches rather than problem-first ones. Many startups are venturing into the LM space, but their value proposition often lacks distinctiveness, leading to an overwhelming number of companies offering similar products, such as Q&A for documents. This approach tends to be ill-conceived as users don't usually ask questions of their documents. There’s a clear indication that more startups should engage with genuine user problems rather than merely incorporating AI for its novelty.
Investment in AI Startups
Investors are often more inclined to back founders who exhibit a genuine understanding of the technology they’re working with and its application in a relevant problem space. A major red flag for investors is startups that seem to be led by the sentiment "LMS are cool, so we should build something with them," rather than a well-defined problem thesis. Trusting the founder’s expertise and intuition about their specific industry often outweighs other concerns.
Emerging Ideas in AI
Beyond the language models, there's a burgeoning space of innovation in image and video AI applications. The image space, in particular, is producing mind-blowing results that have far-reaching appeal, even to non-tech audiences. Voice synthesis, such as the one being developed by 11 labs, is another area with enormous potential, with applications stretching into areas like podcasting.
The Ultimate AI Dream: Harry Potter IRL
A convergence of advancements in AI – from image generation, voice recognition, language modeling, to tts – could bring about an evolution reminiscent of the living pictures in the Harry Potter series. This involves creating digital avatars that can interact in real-time, using the voice, language, and even the image of the person it's based on. Such advancements have the potential to revolutionize how we interact with technology and possibly immortalize personal interactions and memories.
Unpacking AI: Infrastructure Costs, Open Source Dynamics, and the Horizon of Innovations
AI Infrastructure Costs and Transparency
The speaker emphasizes the lack of transparency in large companies regarding the true costs of AI infrastructures. Despite the detailed information on technical specifications, such as TPUs and training durations, the actual financial cost of developing models remains undisclosed. The speaker points out that understanding these costs is vital, especially for startups focusing on unit economics. He even offers a rough estimate on the cost of training some models and mentions the potential discrepancies when calculating these costs.
Open Source Language Models: Advantages and Ethical Concerns
Open source large language models (LLMs) have become a significant talking point in the AI community. Comparing with the image generation models, the speaker notes the success and benefits of open sourcing. However, text generation poses more potential risks. While image forgeries can often be discerned, deceitful or malicious text content is harder to detect. This power, when accessible to individuals, may be misused. Yet, the counterpoint is that democratizing this technology can lead to widespread innovation and prevent monopolies.
Future Innovations in AI
Runway ML is highlighted as an emerging player focusing on video editing with generative techniques. The speaker believes that the future of AI will see advancements in streaming voice recognition, text-to-speech (TTS) systems, and multi-modality applications like video editing and 3D modeling. The speaker also envisions a future where dynamic moving and speaking images, akin to Harry Potter's magical photos, become a reality.
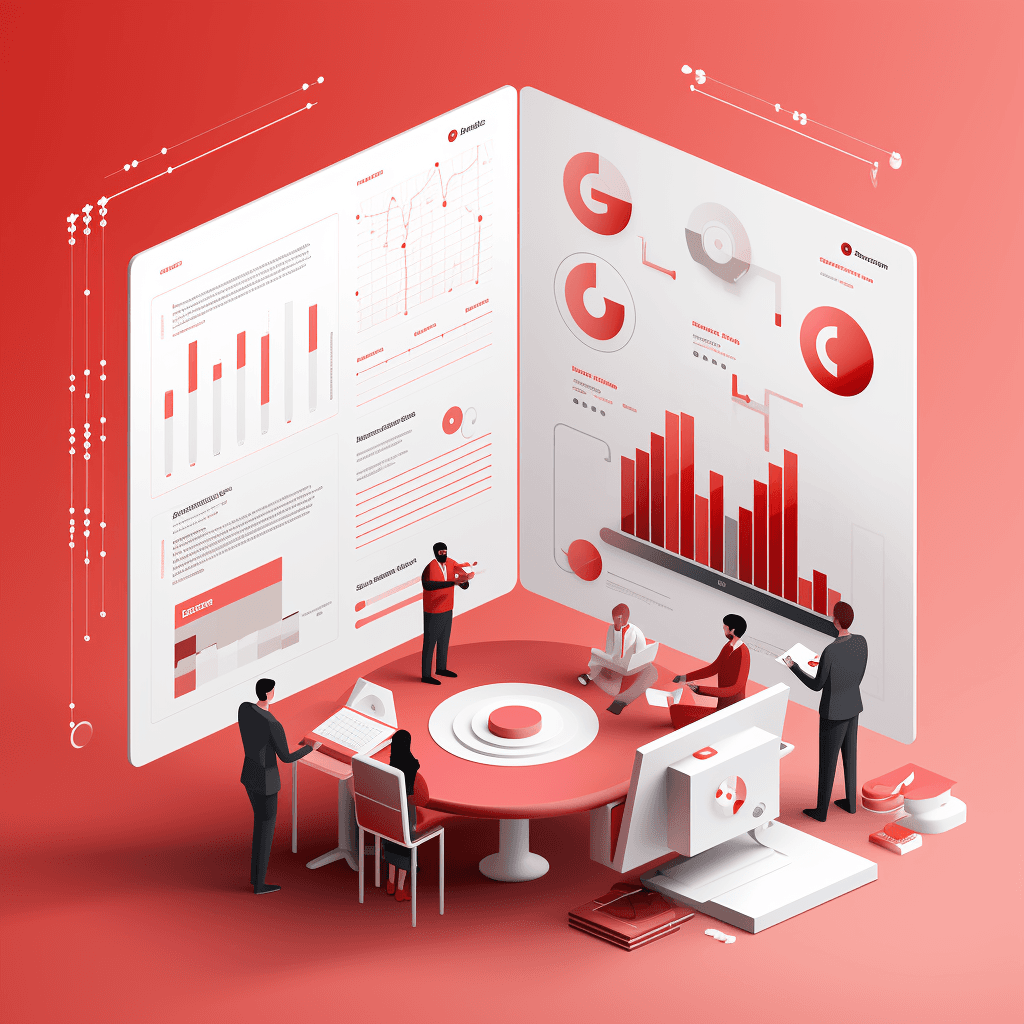
Exam Fraud, Text Generation, and the Art of Detection
AI Models and Text Watermarking:
The conversation delves into the potential harms of unrestricted access to AI language models, emphasizing the need for watermarking to differentiate between human and machine-generated text. The guest advocates for standardized watermarking across various language models for easy detection and understanding.
Examination Fraud and Data Anomalies:
Drawing from personal experience, the speaker sheds light on the manipulation of exam results in the Indian educational system. With the board exams playing a pivotal role in determining college admissions, the conversation unveils data inconsistencies and arbitrary marking. For instance, certain scores like 91 never appeared in any subject in any year, hinting at non-transparent grading manipulation. The speaker mentions an effort in 2013 to scrape and analyze the data, revealing alarming patterns that questioned the legitimacy of the scores.
Comparative Insight:
The discussion transitions to similarities between the examination fraud and financial discrepancies in hedge fund returns. Just as the Indian exam data showed inexplicable patterns, Madoff funds and certain hedge fund returns also exhibited non-normal distributions, suggesting fabricated numbers. Such anomalies serve as "watermarks" that betray human or systemic manipulation. Despite the significant implications, the speaker laments the lackluster response to the exam data inconsistencies in India.

Lightning Round
In the lightning round of the discussion, the guest emphasizes their preferred AI communities and sources. While Reddit isn’t frequently used by them for AI, Twitter emerges as a prime learning platform. AI Pub is particularly highlighted due to its round-up of AI content, which aids in catching up with the latest in the AI world. On the topic of future AI developments, the guest is optimistic about models becoming cheaper and faster, although they believe there might be a cap soon on the quality of AI advancements.
The conversation touches upon practical applications of AI, including a tool that auto-responds to certain messages and drafts answers for more significant ones. While a basic version has been developed, refining it is still ongoing. Another area of interest is an AI system that manages GitHub issues efficiently, even generating PRs to address vulnerabilities.
In wrapping up, the guest stresses the importance of bridging the gap between AI research and end consumers, advocating for more people to delve into AI research papers and watch explanatory content like that of Andre Kapai's YouTube channel. The guest, known as Didi, can be followed on Twitter via "debark_das" and also has a personal website linked from their Twitter profile.