Latent Space Podcast 4/13/23 [Summary] - Segment Anything Model and the Hard Problems of Computer Vision — with Joseph Nelson of Roboflow
Explore Ep.7 with Joseph Nelson on the Segment Anything Model by Meta. Dive deep into Computer Vision's future, the significance of OCR, Image Segmentation, and beyond. #Roboflow #AI
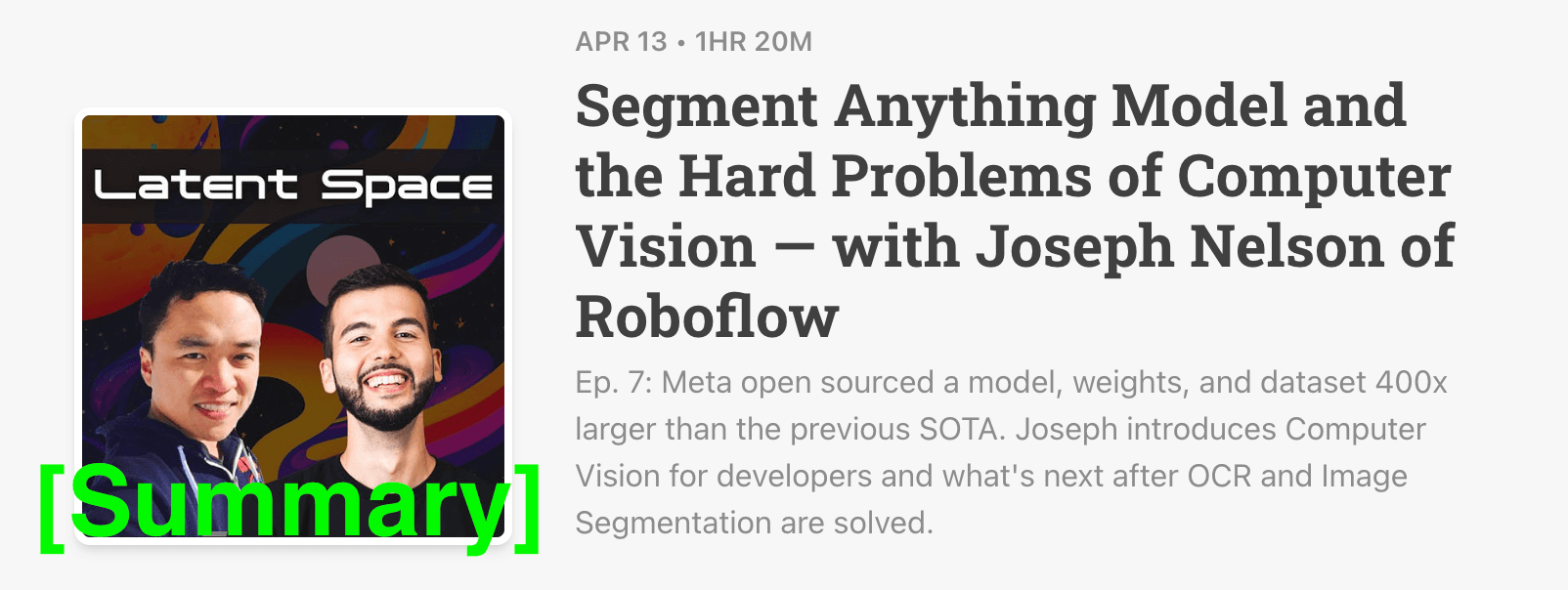
Link to Original: https://www.latent.space/p/segment-anything-roboflow#details
Summary
In this engaging chat, swyx hosts Joseph Nelson, a dynamic individual with a background that spans from economics to politics to tech. Their camaraderie is evident as they discuss past affiliations, with swyx recalling first hearing about Joseph on the Source Graph podcast. Joseph's notable journey includes:
Earning a Bachelor of Economics degree from George Washington University.
Engaging in political activism, notably his creation of "Representing", a tool likened to Zendesk but tailored for Congress to manage their voluminous constituent communications.
Diversifying his experience with roles like a data science instructor at General Assembly and a consultant.
His transition from natural language processing to computer vision, inspired by his ventures at hackathons, specifically his challenges with a chess project at TechCrunch Disrupt in 2019.
The foundation and leadership of Roboflow, his current venture in the AI space.
Joseph exudes pride in his Iowan roots, emphasizing the genuine, caring nature of its people and recounting notable individuals who share his Iowan heritage. He shares a behind-the-scenes look at Roboflow, noting its Des Moines origin, the bond with his co-founder Brad, and their experiences building the company, especially during the Covid-19 pandemic.
The duo hint at exploring the potential applications of computer vision, such as assisting with groceries, and anticipate discussing Roboflow's product 'Segment Anything' in the next part of the conversation.

Origin of Roboflow
The podcast discussed the origin and development of "Roboflow". The journey began with an app called Magic Sudoku, an augmented reality game designed to solve Sudoku puzzles by hovering the phone over it. The creators, after gaining attention for this concept, explored other potential applications. They aimed to add a software layer to the real world, allowing users to interact with objects through a mobile app. They focused on board games initially, starting with Boggle, a game that has players form words from adjacent letter tiles. They then moved onto Chess, developing an app within 48 hours during a hackathon at TechCrunch Disrupt in 2019. This app recognized a chessboard's state and suggested moves. Evan Spiegel, the founder of Snapchat, took an interest in their work at this hackathon. Though they didn't win awards at Disrupt, they gained recognition and subsequently won a $15,000 grant from a Des Moines contest. The podcast also touched upon the confusion around John Papa John, a supporter of the Iowa entrepreneurial scene, who is often mistakenly associated with the pizza brand due to the similarity in name.

Unlocking the Potential of Computer Vision
In a comprehensive discussion about the potential and reach of computer vision, the underlying mission of making the world more programmable through computer vision is emphasized. This technology allows us to interact with our surroundings in a more entertaining, intuitive, and efficient manner. The company aims to provide engineers with the tools, data, and models necessary to develop programmable interfaces swiftly. The scope of computer vision applications is extensive and can range from microscopic tasks to astronomical observations. Several real-world use cases were highlighted:
Recognizing specific ingredients in sushi.
Estimating damages on rooftops.
Monitoring worker safety in workplaces through hardhat detection.
Environmental monitoring to keep track of species count and changes in natural habitats.
Medical applications like early cancer detection by analyzing cell structures.
City planning and traffic management based on real-time vehicle detection.
Home automation projects, such as package arrival notifications or ensuring gates are closed.
Unique DIY projects like a workout machine for a cat powered by computer vision.
Major enterprises like Walmart and Cardinal Health, as well as over 250,000 developers, have employed these computer vision tools, testifying to the technology's adaptability and relevance across sectors. Whether it's enhancing self-driving car datasets or innovatively utilizing computer vision for personal home projects, the overarching message is clear: computer vision holds the key to making the world more interactive, informed, and innovative.

Understanding the Economics of Annotation in Computer Vision
The discourse begins by addressing the challenges of annotation in computer vision. Annotation is often a necessary step when introducing computer vision to a business, especially when an off-the-shelf dataset is unavailable. The speaker highlights the difficulty in estimating the time, effort, and cost involved in annotation.
However, there's optimism in the field. Advancements are ensuring that annotation will become less of a roadblock for computer vision applications. There are emerging models that can recognize items zero-shot, without any annotation. Yet, unique and proprietary data, such as specifics about a product, will still require annotation.
The complexity of the problem and the variance in the scene dictate the number of images required. For instance, recognizing a scratch on a specific part in controlled lighting might need fewer images than recognizing that scratch in varied lighting conditions. There are rough estimates, like using 200 images per class to achieve a 90% accuracy model, but it depends on the specific use-case. The focus is not always on achieving 100% accuracy; sometimes it's about whether a model can outperform a human or at least be a more economical alternative.
Additionally, the value a model provides can sometimes outweigh its accuracy. For instance, in sports analytics, it may be more efficient for a model to track certain movements, even if it's less accurate, due to the cost savings over a human doing the same task.
The conversation then transitions to the topic of computer vision annotation formats. The challenge lies in the myriad ways to describe an object's position in an image. There are multiple formats, such as using the top-left coordinate and bottom-right or using the center of the box with its length and width. Different models and datasets often come with their distinct annotation formats, causing conversion challenges. The speaker mentions the inception of Robo Flow, a tool to convert between these formats, alleviating this common pain point. The discussion also touches upon the worst annotation formats they've encountered, with a mention of a particularly challenging format from a university in China.

Exploring Computer Vision: From Basics to YOLO v8
In a comprehensive discussion on computer vision segmentation, the main themes revolve around the different types of tasks that computer vision can accomplish. These range from straightforward image classification to object detection and advanced tasks like instance segmentation. Instance segmentation, for example, uses polygon shapes for precise area measurements, which can be crucial in applications such as determining the square footage of homes from aerial photos for insurance estimates.
Another interesting point brought up was the key point detection task, which identifies joints or specific points on objects. Visual question answering tasks are also emerging as a game-changer, where an image is presented and the system identifies objects within it, potentially useful for activities like recipe creation based on food items identified in a photo.
A significant portion of the discussion focused on object detection. Here, bounding boxes play a critical role as they provide a versatile solution compared to other methods. Within object detection, different model frameworks have emerged over time. The earlier models, like R-CNN, were relatively slower due to their two-pass method of first identifying potential bounding box candidates and then classifying them.
A revolutionary change came with single-shot detectors, which, as the name suggests, perform object detection in a single pass. The most popular among these is the YOLO (You Only Look Once) family of models introduced by Joseph Redmon at the University of Washington. This model has undergone several versions, with YOLO v5 and its subsequent naming controversy being a notable point. As of the discussion, YOLO v8 stands as the state-of-the-art, marking the continued evolution and sophistication of this framework in the realm of computer vision.

SAM: Revolutionizing Object Segmentation with World Knowledge
Foundational Models and World Knowledge in Computer Vision Discussing the evolution of computer vision, the conversation highlights how recent models incorporate a vast amount of contextual world knowledge. Rather than manually collecting images for training (like for a bus or chair detector), models are now trained on extensive datasets sourced from the internet. The aim is to make the model's corpus as vast as the internet itself. This approach reduces the need for extensive manual annotations. The conversation transitions to talk about distilling the knowledge of large models into smaller, efficient architectures for real-time processing on devices like edge devices.
Segment Anything Model (SAM) Overview SAM, introduced by Facebook as the 'Segment Everything Model', garnered significant attention with 24,000 GitHub stars within its first week. This model is a zero-shot segmentation tool, designed to identify and mask all objects in an image without any prior training on that specific object. What sets SAM apart is its dataset: 11 million images with 1.1 billion segmentation masks. This dataset size surpasses its predecessors, such as Open Images, by a significant margin. SAM facilitates the creation of new applications ranging from background removal to video editing, making object segmentation and recognition significantly more accessible.
Technical Dive into SAM The zero-shot capability of SAM means that it can recognize objects it hasn't been explicitly trained on. SAM's architecture comprises an image encoder and a transformer trained on its massive dataset. When an image is passed through SAM during inference, it first goes through the image encoder to produce an image embedding. This embedding is then passed through a mask decoder, producing multiple candidate masks. SAM can be prompted to focus on particular areas of an image or interact with specific parts, offering a fine-tuned object segmentation process.

RoboFlow's SAM Demonstration: A Glimpse into the Future of Computer Vision
In a multimodal podcast demonstration, the interface of RoboFlow, a computer vision platform, was showcased. Two models were compared: one before SAM (Segmentation and Annotation Model) integration and one post SAM. The primary focus was on image segmentation, particularly on a challenging weld image where two pipes come together. Traditional methods like the Smart Poly, which existed pre-SAM, required multiple clicks to accurately segment the weld, often producing inaccurate selections.
However, with SAM, the precision and efficiency increased dramatically, demonstrating that it could pinpoint details like the weld with significantly fewer clicks. SAM's capabilities were further showcased with various images, including one of two kids with a background brick wall and another with a chihuahua. Its ability to discern and segment specific details (like the eyes of the chihuahua) was underlined.
The broader implications of such advancements were discussed. The ease of creating custom models and IP with RoboFlow was emphasized, suggesting that SAM's introduction has simplified dataset preparation. The hosts also touched upon the imminent potential of GPT-4's multimodality, speculating that features like code generation from imagery and sophisticated OCR using large language models instead of dedicated OCR models might be on the horizon.

Expanding Computer Vision and Roboflow's Journey
In a deep discussion about AI and computer vision, the challenges of expanding the capabilities of AI models like GPT-4 were brought to light. The speaker likened the progression of AI vision to a bell curve, where the center represents common objects and contexts like chairs, cars, and food. This center is steadily growing to encompass less common data and problems, but there are still challenges to face, especially with proprietary information.
A significant portion of the conversation also revolved around Roboflow, a platform to help with computer vision tasks. The speakers touched upon Roboflow's early days, discussing how they started with the idea of translating Stack Overflow to multiple languages, which eventually morphed into the broader Roboflow platform we see today.
Throughout the discussion, there was an emphasis on the importance of both ingesting knowledge and producing content to truly understand and expand in the AI realm. The conversation concluded with some general advice for staying updated in AI, including being genuinely curious, engaging with various sources, and participating actively in the community.